Data science abroad: Everything you need to know!
Dr. Samik Raychaudhuri, Head of Data Science and Infrastructure at Auquan, shares essential data science skills, program details, and practical strategies you'll need on your data science journey.
Watch Webinar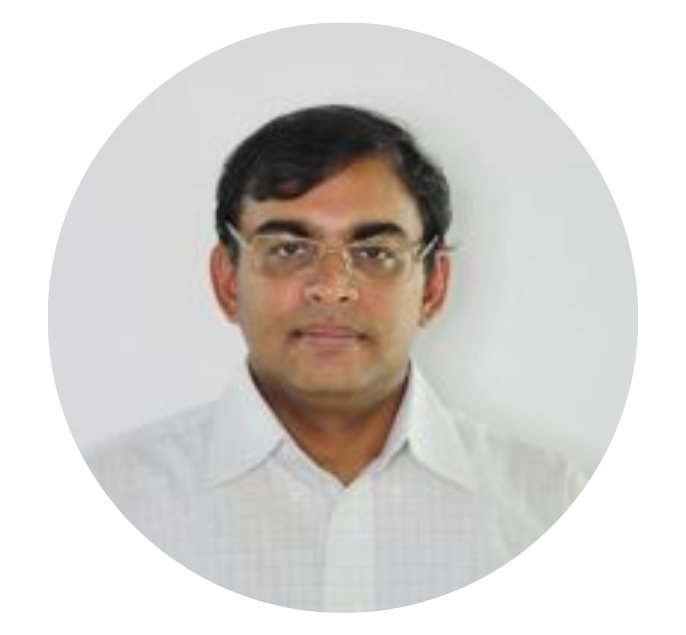
About the Speaker:
-
Samik Raychaudhuri, based in Bengaluru, India, currently serves as the Head of Data Science and Infrastructure at Auquan.
-
Drawing from past experiences at Oracle, Quick Ride, and [24]7.ai, he holds a Ph.D. from the University of Wisconsin-Madison.
-
With expertise in Operations Research, Predictive Analytics, and more, Samik offers valuable contributions to the industry, bringing a wealth of insights and knowledge.
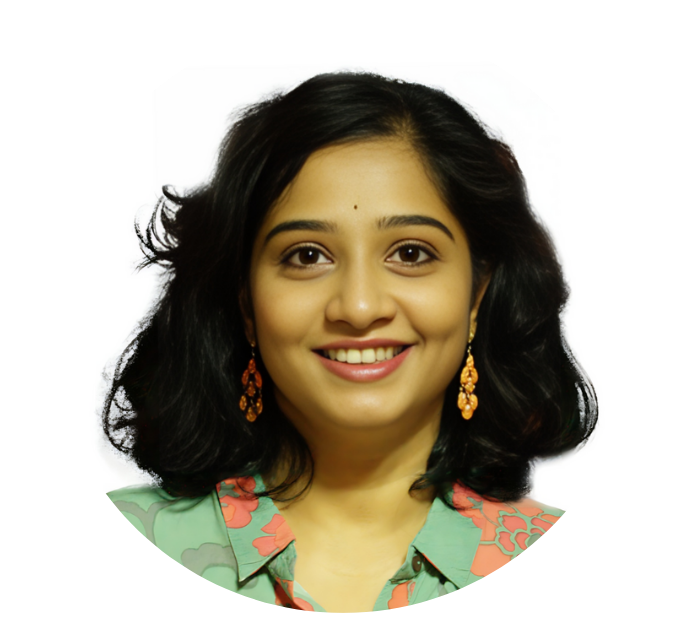
Pavithra Srinivasan,
Founder & Product Director, Galvanize
M.S., in Electrical Engineering, Stanford university
About the Host:
-
Pavithra has graduated from Stanford University with a 100% Scholarship in her domain.
-
She returned to India with a vision to revamp the Test Preparation space with a more personalized study based program and started Galvanize.
-
Pretty much all of the courses we offer have been personally worked on by Pavithra – giving it that elite Stanford touch.
Watch the webinar now to explore data science with Samik Raychaudhuri.
Session conducted on Sunday, November 26, 2023
Key Highlights of the session:
1. Preparation and Prerequisites:
- Emphasized the importance of a strong foundation in mathematics, statistics, and programming for aspiring data science students.
2. What’s in the Program?
- Highlighted the key concepts covered in a two-year master’s program, including statistical analysis, machine learning, and potential areas of specialization.
3. Skills and Experience:
- Addressed ways to gain data analytics skills without experience, emphasizing online courses, personal projects, and networking opportunities.
4. Data-related Fields:
- Clarified the distinctions between data analytics, data science, machine learning, and business analytics to help students understand their focus areas.
5. Admission Strategies:
- Provided insights into standing out with a low CGPA, limited programming experience, and increasing chances of admission for those with no work experience.
6. Global Career Opportunities:
- Discussed the scope and opportunities abroad for data science, & considerations for leaving a top software industry in India for a master’s abroad.
Our students who got admits for Data science program.
Get your profile evaluated now to explore more about the Data science program!
Explore Now!Sneak- Peak of the Q&A session!
1. What prerequisite one must have to pursue a master’s in data science?
1. Educational Background: A Bachelor’s in science or engineering is a common prerequisite. Computation skills are crucial for a data science Master’s, so familiarity with coding languages, especially Python, is essential.
2. Business Understanding: Knowing the business areas where data science is applied is vital because data science is fundamentally an applied field, particularly in business contexts..
3. Broad Applicability: Data science is applicable in various areas, and understanding these application areas is key to preparing for a Master’s in Data Science. The intent to apply acquired skills to solve real-world business problems or research challenges is an essential aspect of a data science mindset.
4. Analytical Skills: Strong analytical and logical thinking, regardless of the educational background, is crucial. Having an interest in spotting patterns or trends in large datasets is beneficial for success in data science.
5. Diverse Backgrounds: While a science or engineering background is common, students from diverse educational backgrounds, like a BBA, commerce with strong math or statistical skills can also succeed in data science.
2. What concepts does one learn under the two-year master’s program in data science?
Practical Skill Emphasis:
Data science programs prioritize real-world skills, integrating statistics, mathematics, and computer science to equip students with the practical expertise needed in the industry.
Specialization Opportunities: Students can specialize in unique areas such as statistical analysis, advanced mathematics, or CSE, enabling them to develop expertise in specific aspects of data science.
Computational Proficiency: Proficiency in computation, coupled with knowledge of computer architecture & programming methods, is emphasized to prepare data scientists for handling complex datasets.
3. What would be the ROI if we get admitted as a data scientist?
The cost of pursuing a Master’s degree in the US can range from $25,000 to $50,000 annually, covering tuition and living expenses. Investing between $50,000 to $120,000 for a two-year program is common. Costs vary across countries; Singapore is more affordable, but job placement can be challenging. Germany, with potential language barriers, offers tuition coverage in top institutes. Considering return on investment (ROI), starting salaries for US data scientists range from $100,000 to $120,000 per year. Adopting a frugal lifestyle can help manage expenses and potentially pay off education loans. Scholarships may also be available based on your application. Despite the competitive job market, the lucrative starting salaries make data science a sought-after field, promising a strong foundation for a successful career.
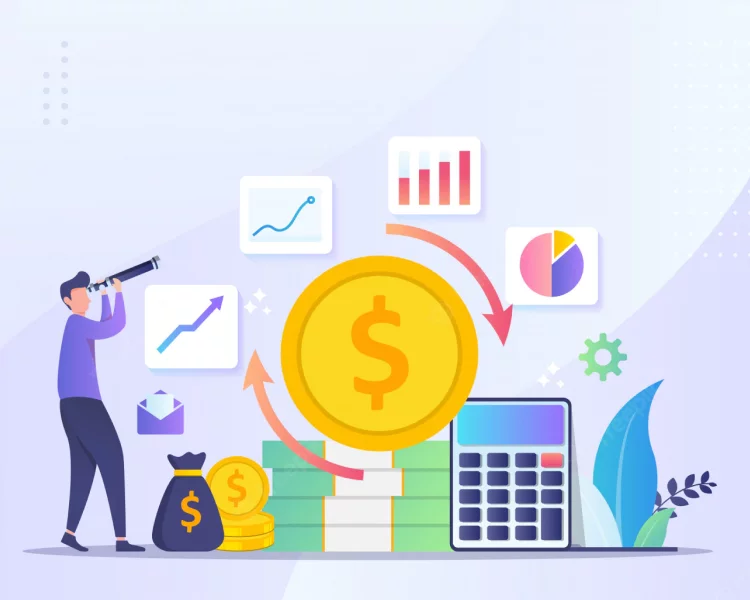
4. Which country apart from the USA should be recommended for Data Science and why?
-
Selecting the right destination for pursuing studies in data science involves careful consideration, especially given the uncertainties in the legal and immigration frameworks of certain countries. While the United States (US) remains a key option, the recent fluctuations in its legal and immigration landscape make it prudent to adopt a portfolio approach. Exploring universities not only in the US but also in other geographical areas helps diversify options.
-
Canada stands out as a favorable choice, supported by the presence of influential researchers in machine learning, particularly in cities like Vancouver and Toronto. Despite ongoing issues, Canada maintains its appeal. Additionally, the United Kingdom (UK), Australia, and Singapore emerge as promising alternatives. Europe, while offering quality programs, may present a language barrier, unlike English-speaking countries such as the UK, Australia, and Singapore. Considering career prospects is crucial when applying to data science programs. It’s essential to recognize the vast potential for careers in data science and analytics both within and outside one’s home country.
-
India, for instance, is experiencing rapid growth with numerous opportunities. In the US, the maturity of certain regions varies. For instance, the Bay Area is highly developed, offering numerous opportunities but with intense competition. Exploring emerging cities within the US and applying the same principle to other countries helps identify pockets where maturity and competition levels differ. This strategic approach ensures a well-rounded exploration of opportunities, making it easier to find a balance between competitiveness and accessibility in different locations.
5. How do I transition to a career change from Non-CS Engineering to Data Scientist?
-
Changing Landscape in Data Science Careers: Many businesses now seek professionals who can apply existing models rather than creating new ones. This change emphasizes a blend of data science and engineering skills.
-
Transition Path for Non-Tech Backgrounds: To make a smooth transition into data science, consider a path that involves progressing from data engineering to ML (Machine Learning) engineering and finally to data science. Data engineering involves handling and organizing data, ML engineering focuses on implementing ML models in real-world scenarios, and data science centers around building and innovating these models.
-
Steps for a Career Change: Start by becoming comfortable with coding and algorithms. Take advantage of free online courses that cover coding and data science fundamentals. Gain practical experience by working on small projects within your current organization. Consider taking on freelance projects. Numerous websites offer paid freelance opportunities to apply your skills.
-
Learn through Collaboration: Engage in contest platforms like Kaggle. Form a peer group with one or two experienced individuals in data science to collaborate and learn faster. Look for internships in data science, whether paid or unpaid, to gain experience and enhance your resume.
6. What is the main difference between Data Analytics, Data Science, Machine Learning and Business Analytics?
-
Data analytics and business analytics are closely related but have different goals and approaches. They mainly use prescriptive analytics, focusing on analyzing data to give useful insights for decision-making. Professionals in these fields use tools like statistics, econometrics, and visualization to present results that guide business decisions.
-
In contrast, data science and machine learning are about predictive modeling. People in these fields create models to predict future business outcomes. They not only use statistical methods but also rely on mathematical principles like linear algebra and combinatorics. Knowledge of computer science and advanced techniques like deep learning and generative AI is crucial.
-
To simplify, data analytics and business analytics concentrate on presenting and making decisions for businesses. On the other hand, data science and machine learning go deeper into predicting future outcomes using a wide range of mathematical, statistical, and advanced computational techniques.
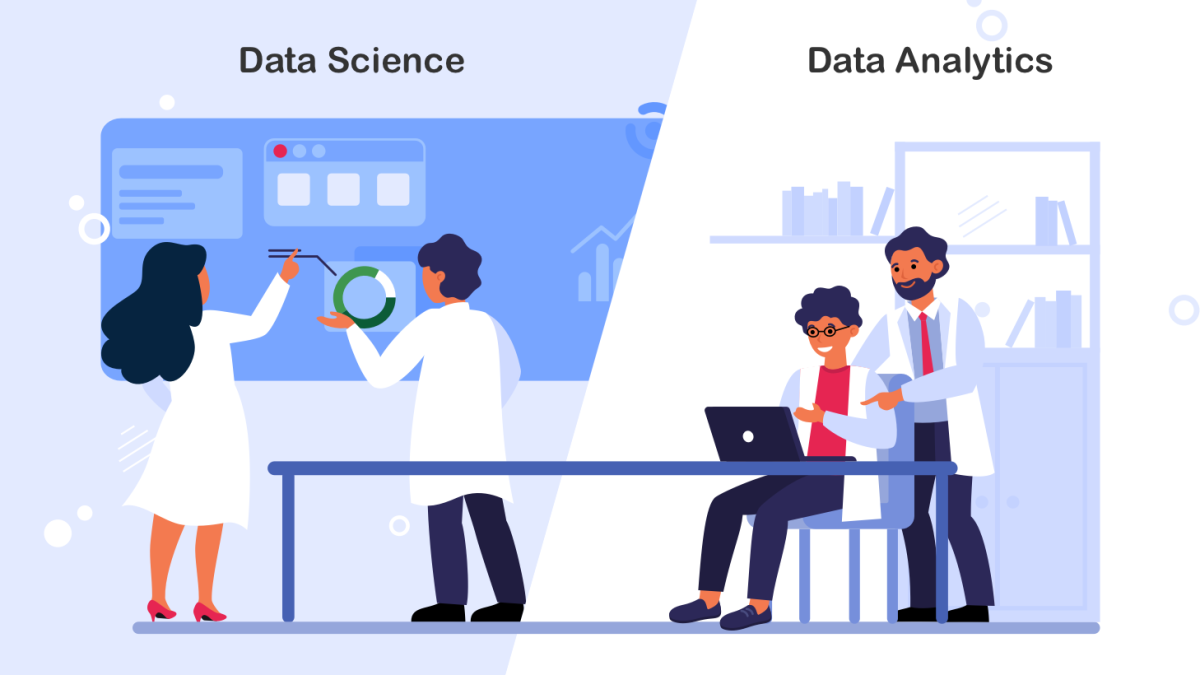
Meet the Team!
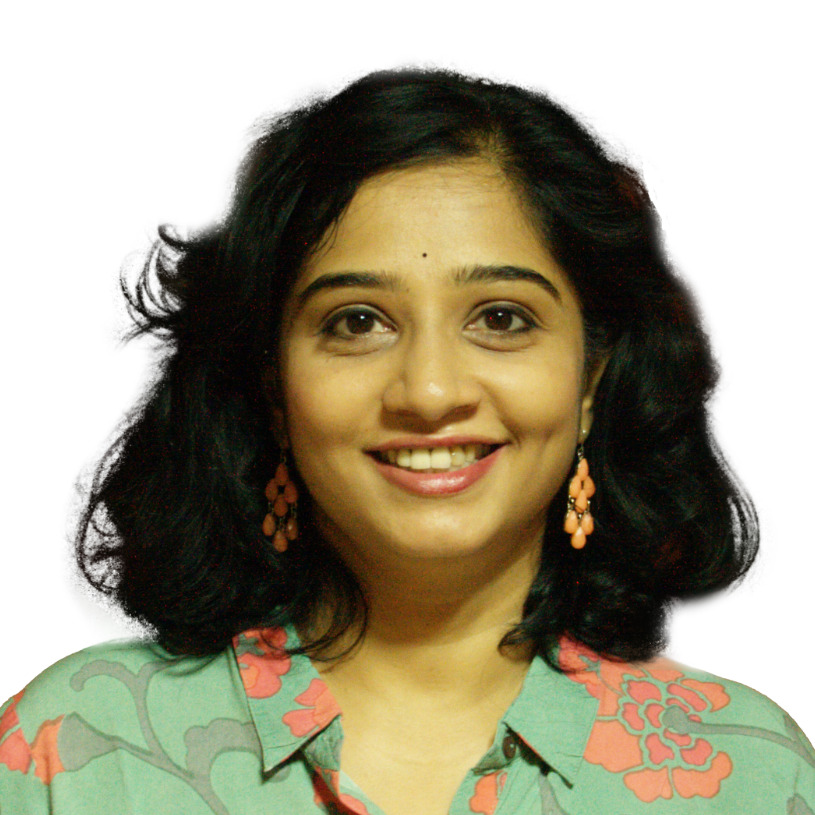
Pavithra Srinivasan
Co-founder & Head of Customer Experience
M.S., Stanford University
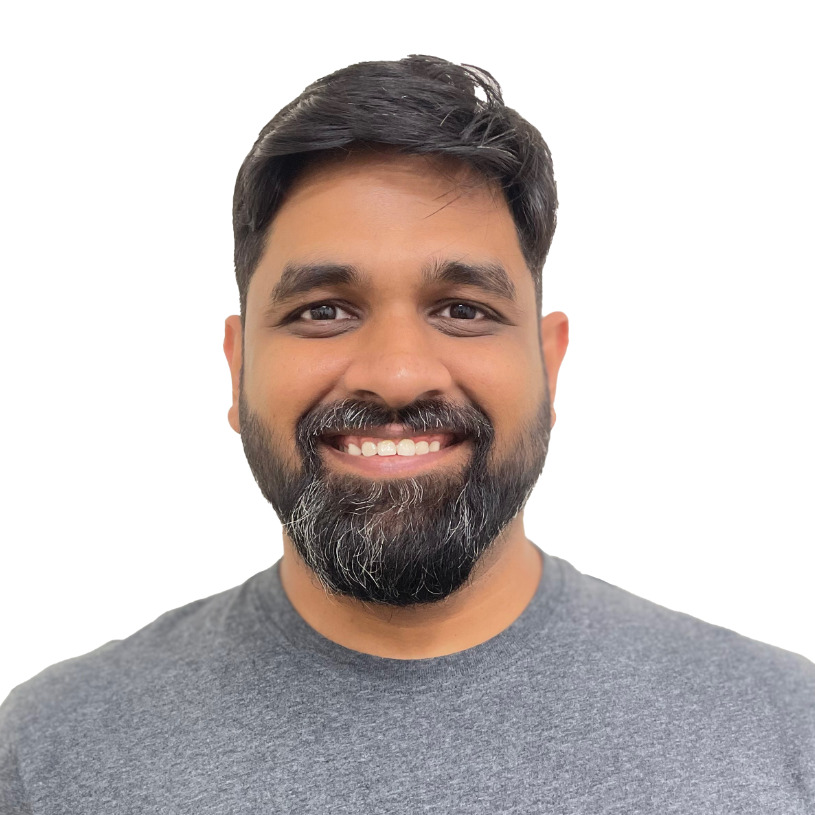
Sriram Venkatraman
Co-founder & Chief Product
Architect
B.Tech., IIT Madras
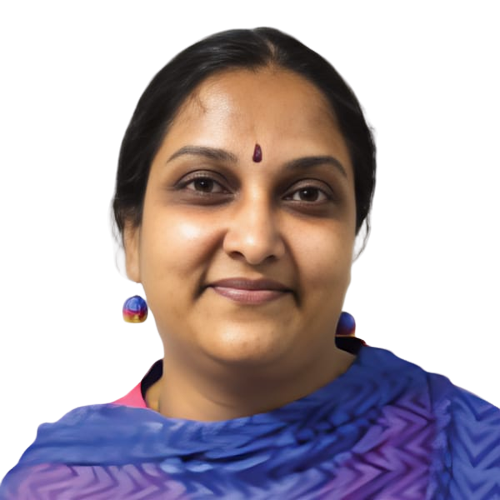
Sowmya
Subramaniam
Head of Academics
M.Sc, Maths , IIT Madras
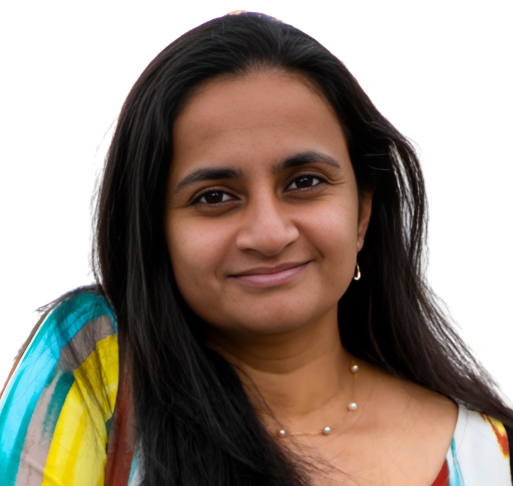
Jayanthy
Ramakrishnan
Head of Admissions